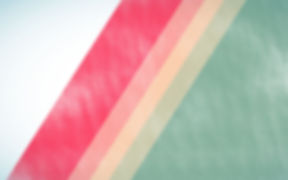
EASTERN
Towards Micro-video Understanding by Joint
Sequential-Sparse Modeling
ACM MM 2017

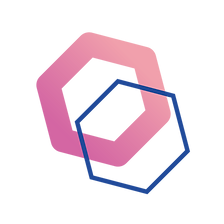
Like the traditional long videos, micro-videos are the unity of textual, acoustic, and visual modalities. These modalities sequentially tell a real-life event from distinct angles. Yet, unlike the traditional long videos with rich content, micro-videos are very short, lasting for 6-15 seconds, and they hence usually convey one or a few high-level concepts. In the light of this, we have to characterize and jointly model the sparseness and multiple sequential structures for better
micro-video understanding. To accomplish this, in this paper, we present an end-to-end deep learning model, which packs three parallel LSTMs to capture the sequential structures and a convolutional neural network to learn the sparse concept-level representations of micro-videos. We applied our model to the application of micro-video categorization. Besides, we constructed a real-world dataset for sequence modeling and released it to facilitate other researchers. Experimental results demonstrate that our model yields better performance than several state-of-the-art baselines.

We first leverage three independent LSTMs to parallelly characterize the sequential structures of three modalities. We then project their outputs into a common space by three mapping functions. After that, we input the three projected vectors with the same length into a convolutional neural network to learn their sparse conceptual representations, whereby the K filters serve as the k atoms in a dictionary. We finally adopt a classifier for further classification tasks.

We would like to thank the anonymous reviewers for their valuable comments. This work was supported by the Joint NSFC-ISF Research Program (No.61561146397), jointly funded by the National Natural Science Foundation of China and the Israel Science Foundation. It is also supported in part by the National Basic Research grant (973)(No.2015CB352501) and the One Thousand Talents Plan of China (No.11150087963001).
-
LSTM+DL: LSTM+DL.py
-
LSTMs: LSTMs.py
-
CONV: CONV.py
-
TMDL: TMDL.rar
-
DMDL: DMDL.rar
-
TRUMANN: TRUMANN.rar
-
EASTERN: EASTERN.py

Paper
Slides
Coming Soon
Sequence data set : It is designed for deep learning model based on LSTM network.
visual modality: visual.h5
audio modality: audio.h5
text modality: text.h5
Non-sequence data set: It generated by averaging all the sequences of each modality.It contains 4096-D visual feature, 512-D audio feature, and 100-D text feature.
feature: feature.mat
label: label.cvs
​